期刊信息
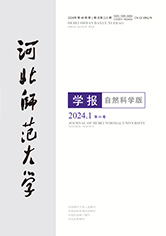
- 刊名: 河北师范大学学报(自然科学版)Journal of Hebei Normal University (Natural Science)
- 主办: 河北师范大学
- ISSN: 1000-5854
- CN: 13-1061/N
- 中国科技核心期刊
- 中国期刊方阵入选期刊
- 中国高校优秀科技期刊
- 华北优秀期刊
- 河北省优秀科技期刊
带参数优化的随机森林变压器油中溶解气体浓度预测
- (国家电网内蒙古东部电力有限公司 电力科学研究院,内蒙古 呼和浩特 010000)
-
DOI:
10.13763/j.cnki.jhebnu.nse.202202016
Concentration Prediction for Dissolved Gases in Transformer Oil Based on Random Forest with Parameter Optimization
摘要/Abstract
变压器作为电网运行中的关键设备,其油中溶解气体浓度预测一直是研究热点.随机森林算法作为一种泛化能力很强的集中机器学习算法,算法性能与算法参数选择存在密切影响关系.现已报道的利用随机森林算法解决变压器油中溶解气体浓度预测问题的研究,忽略了对参数取值问题的讨论.利用遗传算法对随机森林算法中的5个重要参数进行优化,将优化结果代入随机森林算法,以乙炔为例,所得油中溶解气体预测相对误差为2.66 %,结果小于不进行参数优化时的相对误差(3.24 %),算例结果验证了模型的有效性.
Transformer is a the key equipment in power network operation, and the concentration prediction for dissolved gas in transformer oil of is always a hot research issue. Random forest algorithm is a concentrated machine learning algorithm with strong generalization ability, which has close relationship with its key parameters. By using the random forest algorithm, the existing research on the concentration prediction for of dissolved gas in transformer oil ignores the effect of parameter selection on the algorithm. Based on the parameter selection, the paper uses the genetic algorithm to optimize the value of five important parameters of the random forest algorithm. The optimization results are put into the random forest algorithm, and the error of acetylene gas prediction is less than 2. 66 %, which is better than the case without parameter optimization(3. 24 %). The case calculation results verify the effectiveness of the proposed model.
关键词
参考文献 9
- [1] 周湶,孙超,安文斗,等. 基于云推理及加权隐式半Markov模型的变压器故障预测[J]. 高电压技术,2015,41(7):2268-2275. doi: 10.13336/j.1003-6520.hve.2015.07.021 ZHOU Quan,SUN Chao,AN Wendou,et al. Transformer Failure Pre-diction Based on Cloud Reasoning and Weighted Implicit Semi-markov Model[J]. High Voltage Engineering,2015,41 (7):2268-2275.
- [2] 蔡胜伟,陈江波,邵苠峰,等. 电力变压器用天然酯绝缘油中特征气体溶解特性[J]. 高电压技术,2017,43(8):2608-2613. doi: 10.13336/j.1003-6520.hve.20170731022 CAI Shengwei,CHEN Jiangbo,SHAO Minfeng,et al. Dissolution Properties of Characteristic Gases in Nature Ester Insulating Oils for Power Transformer[J]. High Voltage Engineering,2017,43(8):2608-2613.
- [3] 周湶,王时征,廖瑞金,等. 基于 AdaBoost 优化云理论的变压器故障诊断方法[J]. 高电压技术,2015,41(11):3804-3811. doi: 10.13336/j.1003-6520.hve.2015.11.039 ZHOU Quan,WANG Shizheng,LIAO Ruijin,et al. Power Transformer Fault Diagnosis Method Based on Cloud Model of AdaBoost Algo-rithm[J]. High Voltage Engineering,2015,41 (11):3804-3811.
- [4] 陈新岗,杨定坤,谭昊,等. 基于峰度的变压器油中特征气体拉曼光谱分析[J]. 高电压技术,2017,43(7):2256-2262. doi: 10.13336/j.1003-6520.hve.20170628022 CHEN Xingang,YANG Dingkun,TAN Hao,et al. Raman Analysis on Characteristic Gases in Transformer Oil Based on Kurtosis[J]. High Voltage Engineering,2017,43(7):2256-2262.
- [5] MICHEL D.A Review of Faults Detectable by Gas-in-oil Analysis in Transformers[J]. IEEE Electrical Insulation Magazine.2002,18(3):8-17.doi:10.1109/MEI.2002.1014963
- [6] 徐肖伟,李鹤健,于虹,等. 基于随机森林的变压器油中溶解气体浓度预测[J]. 电子测量技术,2020,43(3):66-70. doi: 10.19651/j.cnki.emt.1903364 XU Xiaowei,LI Hejian,YU Hong,et al.Concentration Prediction of Dissolved Gases in Transformer Oil Based on Random Forest[J]. Electronic Measurement Technology,2020,43(3):66-70.
- [7] 韦东. 基于随机森林算法的知乎优质答案推荐研究[D]. 哈尔滨:黑龙江大学,2021. WEI Dong.Research on High-quality Answer Recommendation in Zhihu Based on Random Forest Algorithm[D]. Harbin:Heilongjiang University,2021.
- [8] 刘兴,王艳,纪志成. 基于随机森林的风电功率短期预测方法[J]. 系统仿真学报,2021,33(11):2606-2614. doi: 10.16182/j.issn.10047 LIU Xing,WANG Yan,JI Zhicheng. Short-term Wind Power Prediction Method Based on Random Forest[J]. Journal of System Simulation,2021,33(11),2606-2614.
- [9] 刘晓明,王新,徐慧. 基于多目标随机森林的煤层厚度同步预测方法[J]. 计算机工程与设计,2021,42(4):1116-1121. doi: 10.16208/j.issn.1000-7024.2021.04.031 LIU Xiaoming,WANG Xin,XU Hui. Synchronous Prediction Method for Coal Thickness Based on Multi-objective Random Forest[J]. Computer Engineering and Design,2021,42(4):1116-1121.